Applications to Additive Manufacturing
Calculate the following as a function of material chemistry and temperature:
Application Examples
Improving Finite Element Modeling with CALPHAD Data
The accuracy of finite element (FE) simulations depends in part on the material property data used by the code. For example, to predict the size, shape, and temperature of the laser melt pool, FE codes need the latent heat and heat capacity of the material. Handbooks are a common source of data, but these typically do not have temperature-dependent data for industrial alloys or data for novel materials. Temperature-dependent data can be calculated using Thermo-Calc for specific chemical compositions of interest.
These figures show a comparison of handbook values and CALPHAD calculated values of Cp (top) and ΔH (bottom) for a 316L Stainless Steel (recalculated from Smith et al. Comput. Mech. 2016).
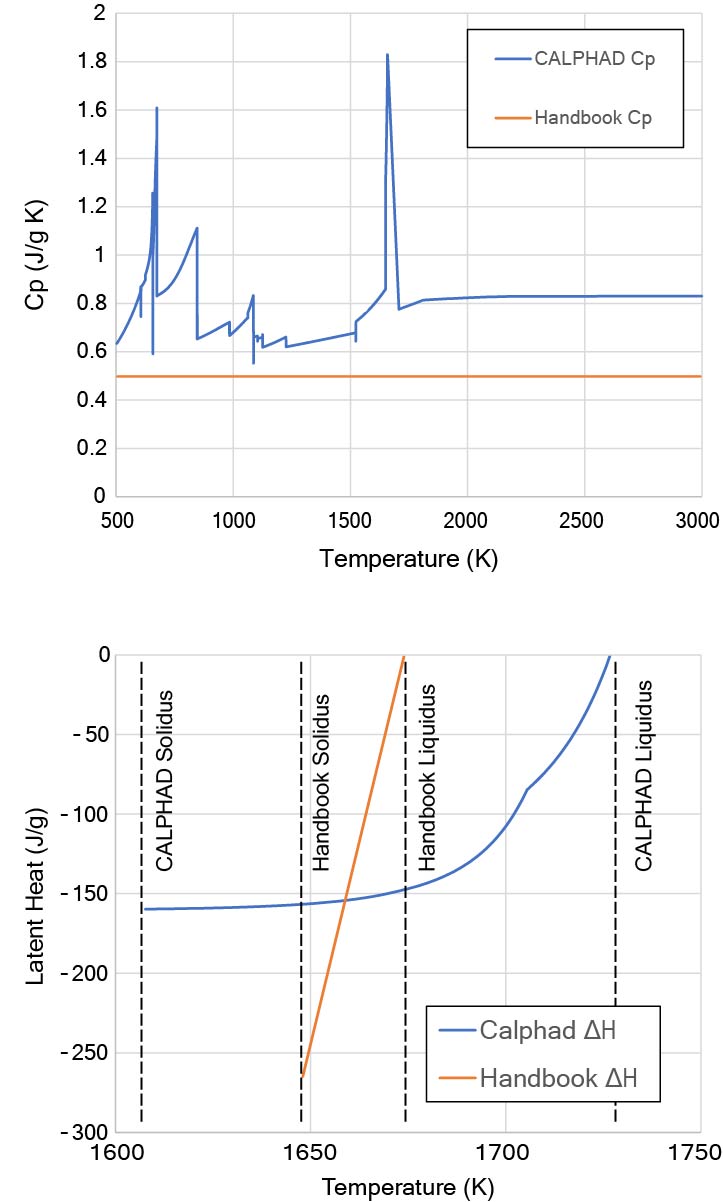
Capturing the Effects of Rapid Solidification
Rapid solidification, typical of additive manufacturing processes, can lead to solute trapping where solute may be incorporated into the solid phase at a concentration significantly different from that predicted by equilibrium thermodynamics. These effects influence microsegregation, solidification morphology, and the emergent microstructure length scales.
The Scheil Solidification Simulation Calculator in Thermo-Calc includes a model that considers solute trapping in the primary phase for calculations that have a high solidification speed, the Solute Trapping model. The model is based on the main assumption that the primary phase is the only dendrite forming phase within which solute trapping is induced by high solidification speed. The resulting solute trapping also affects composition and amount of other solid phases.
This figure shows a Scheil Solidification Simulation for an Al-Si-Mg alloy using the Solute Trapping model compared with a Classic Scheil Solidification Simulation and equilibrium. The plot shows that the Solute Trapping model is closer to equilibrium as the solidification progresses.
Predicting Optimal Post-build Heat Treatments
During precipitation heat treatment of additively manufactured 625 parts, the deleterious delta phase can precipitate faster than in wrought 625, leading to degradation of properties. Zhang et al (Acta Mater. 2018) have used Thermo-Calc and the Precipitation Module (TC-PRISMA) to predict the precipitation kinetics of the delta phase for nominal feedstock compositions, as well as the compositions measured at dendrite boundaries.
Both simulations, shown in the figures, predict that a stable MC carbide forms, followed by some gamma double prime (γ″). Delta (δ) phase then forms at the expense of the γ″. Because of segregation during solidification, γ″ and δ phase both precipitate much more quickly in the interdendritic region due to the increased Nb and Mo. Delta phase is predicted to start forming around 1 hour, compared with 10 hours for the wrought material. These calculations give insight to the reason why the conventional stress-relief heat treatment is not suitable, and can be used to identify a suitable heat treatment to both homogenize and stress relieve the part, while avoiding deleterious phases.
Predicting Melt Pool Size
Knowing how the process parameters affect the melt pool size is key in additive manufacturing to get a robust and homogeneous build. With the Additive Manufacturing Module in Thermo-Calc, you can simulate the steady state behavior of the melt pool for any material and process parameter. Grange et al. performed a range of single-track experiments on Inconel 738LC with a composition of Ni-15.8 Cr-8.6 Co-2.7 W-3.3 Ti-3.6 Al-1.8 Ta-1.8 Mo-0.04 Fe-0.8 Nb-0.002 Mn-0.1 C Mass % and measured the width and depth of the melt pool.
The single-track experiments with lowest (0.24) and highest (0.47) ratio between Power and scan speed (P/v) were chosen to optimize the parameters for the double ellipsoid heat source, also known as Goldak heat source. Many simulations were run, with varying Heat Source parameters, until good agreement with Grange’s melt pool dimensions were obtained. A linear relation for each Heat source parameter was then applied, as a function of P/v, to simulate all cases in Grange et al.
The comparison between experimental and predicted melt pool sizes are shown in the figures here. As seen, the optimized parameters give a very good agreement between predicted and experimental melt pool dimensions for all process cases, although it was only optimized using 2 of them.
Powder Bed Fusion
Additive Manufacturing Module
The Additive Manufacturing Module is an Add-on Module in Thermo-Calc that allows users to model the Powder Bed Fusion process. Special focus has been to have a unified treatment of alloy dependent material properties and process parameters when solving the multiphysics problem of a moving heat source that melts and solidifies metal powder.
Learn More about Applications to Additive Manufacturing
Accelerated Design of Printable Superalloys for Additive Manufacturing: CALPHAD- and ICME-based Approach
Thermodynamic and Kinetic Simulations on Joining and Additive Manufacturing Processes for an ICME Framework
Applications of CALPHAD-based tools to additive manufacturing
Development of Non-Equilibrium Thermodynamic Tools for Additive Manufacturing
Degradation of AlSi10Mg powder during laser based powder bed fusion processing
An integrated computational materials engineering-anchored closed-loop method for design of aluminum alloys for additive manufacturing
Application of finite element, phase-field, and CALPHAD-based methods to additive manufacturing of Ni-based superalloys
Additive Manufacturing of 17-4 PH Stainless Steel: Post-processing Heat Treatment to Achieve Uniform Reproducible Microstructure
Toward an integrated computational system for describing the additive manufacturing process for metallic materials
Effect of the Process Atmosphere Composition on Alloy 718 Produced by Laser Powder Bed Fusion
Modelling of additive manufacturability of nickel-based superalloys for laser powder bed fusion